Bank technologists have warmed to the idea of using artificial intelligence and machine learning technology in many areas — lending, anti-money- laundering compliance, and collections among them.
But banks also face a “black box” problem when they try to implement AI. They can’t run an AI model unless they can prove to bank executives and regulators that it’s fair, safe and has “explainability” in which the reasoning behind decisions is plainly spelled out.
“Financial services has been largely untouched by machine learning except for fraud,” said Douglas Merrill, CEO and founder of ZestFinance, which provides machine learning-based software for lending and for compliance with anti-money-laundering rules. “It’s not like financial services firms are dumb. Every large financial institution has someone in a lab working on machine learning, and all those people know these new algorithms are much better than the old ones. But none of them get into production,” primarily because of regulatory issues like explainability.
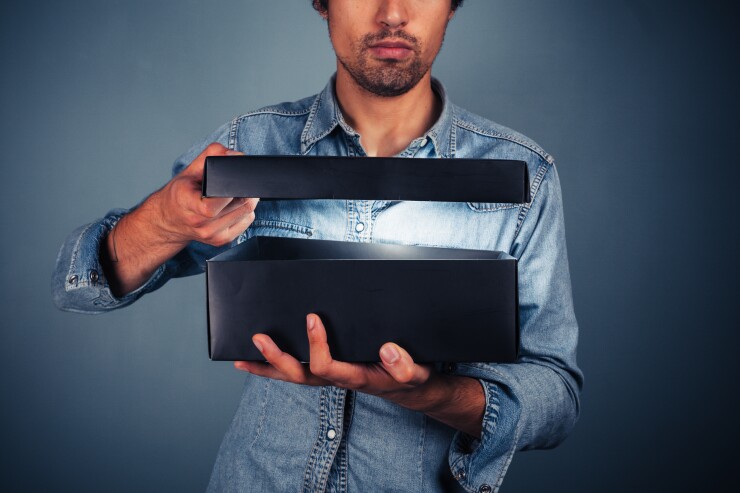
Bank technologists agree.
“The biggest fear I have with applying machine learning to anything is, I don’t think the regulators are there yet,” one said on condition of anonymity. “I can use it to detect anomalies. But when it comes to how we treat people or how we apply policy to customers, that’s where regulators have a tough time. They’re used to seeing a flowchart — if you use this condition, you get this.”
But is there a way beyond this issue? Can AI ever live up to its potential for financial services?
Unintended bias
The need for explainability comes up most in lending.
The idea of making loan decisions in a black box is horrifying to consumer advocates and regulators alike. What if the black box is considering a data point in its decision that correlates strongly with ethnicity? What if the black box mimics discriminatory human behavior by unconsciously picking up cues such as people who belong to a certain golf club tend to be a good credit risk?
“The use of any technology poses risks, and the use of AI and machine learning in loan underwriting is no different,” said David Berglund, senior vice president of artificial intelligence at U.S. Bank. “We are mindful of the need to limit bias, ensure fairness and maintain controls.”
But some argue that for all of AI's potential pitfalls, the current system without AI is worse.
Paul Gu, co-founder of Upstart, an online lender that white-labels its AI-based loan platform to Customers Bancorp, BankMobile and others, strongly objects to the idea that the use of alternative data and AI leads to discrimination.
“The traditional ways of underwriting are about as unfair as it gets,” he said. “If someone is worried about only lending to well-off people, hey, do you use income? What is the definition of being well off? A high FICO score. What do you think is the percentage of people in a certain race who have a high FICO score? If you compare that number to the percent of people at top U.S. educational institutions, one of those numbers is way better than the other. It doesn't go against education.
“We're able to dramatically improve access to credit,” he said. “Our mission is, every person who would actually pay back a loan, we want to give them a loan at the lowest possible rate.”
Old-school explainability
Berglund likens explainability to a student showing her work on a test.
“You may have found the ‘right’ answer, but can you do it consistently over time and with new model inputs?” he said.
Today, consumers who are declined for a loan are given “adverse action” codes or reasons for the denial. Sometimes the reason codes come straight from the credit bureaus.
“Our data shows that almost 80% of those reasons are false,” Merrill said. “The credit bureau did their best to come up with reason codes, but banks’ underwriting includes more than the credit bureaus’ data, so it’s quite unlikely that they happen to overlap.”
Gu likewise criticizes the status quo.
“The adverse action in effect at most lenders was designed for a world with really binary underwriting, where the underwriting was, if your credit score is here, it's good, if your debt to income ratio is here, it's good,” he said.
The adverse action codes generated by older lending systems often aren’t even true, let alone fixable, he said. Sometimes people are told they can’t get a loan because they don’t have enough debt, for instance.
Upstart has been controversial because it considers data about an applicant’s education — degrees obtained, schools attended, areas of study and years of graduation — alongside employer and occupational attribute data, data from the loan application itself and traditional credit bureau data in its models. It received a no-action letter from the Consumer Financial Protection Bureau that allows it to continue what it’s doing without fear of reprisal, in return for sending the agency detailed information about all the loan applications it receives, approves and declines.
Gu says Upstart has full explainability.
“If you're going to turn someone down, you should give them a useful explanation of why you turned them down,” Gu said. “We do that; that's a solvable problem. You have a model and it's complicated, but complicated math can solve complicated math. Our model helps you surface reasons to the consumer that are in our view better than traditional systems.”
Similarly, Scott Zoldi, chief analytics officer at FICO, said the scoring company’s AI-based AML software can generate a story as well as a reason code for each potential money laundering incident it flags.
“If every decision came with not only reason codes but a human experience story, the customer could explain why we stopped the transaction, there’s a whole different level of explainability,” he said. “It’s not enough that the machine learning model is explainable in some academic sense. There has to be someone on the other side of the phone who can speak to me in human language about why my transaction got stopped. That’s where the evolution is occurring.”
New forms of explainability
Academics have created techniques for drawing explainability out of mathematical models used in machine learning. One called LIME came out in 2016. Another popular technique that came out last year is called SHAP, an acronym for SHapley Additive exPlanations. ZestFinance and underwrite.ai both use SHAP.
Underwrite.ai has been generating fully explainable models since 2014, according to CEO Marc Stein. “It’s not a new thing.”
“For any given decision, we can interrogate the model and say, what were the attributes that led you to the conclusion you reached?” he said.
If underwrite.ai’s model declines a loan application, it will provide a ranking of the most important data points that factored into the rejection. One might be a two-year delinquency. Another might be an adverse public record.
“It isn’t a rule set. It isn’t that we said, ‘Decline anything with public records,’ ” Stein said. “But the model has learned that where there are occurrences of high delinquencies and public records, the probability of an outcome being good is quite low. It’s easy to map those to the proper adverse-action reasons so it can be correctly explained to the consumer.”
Berglund said he’s looked at several vendors’ explainability solutions. He won’t comment on any specific vendor, but he sees promise in all of them.
“We are excited by the increasing investment being poured into this market of explainable AI,” he said.
Convincing the regulators
In private conversations, bankers often say that while publicly bank regulators express openness to the use of AI, local examiners often don’t understand the technology and will object to it.
Zoldi said several bank customers have asked him to explain to local regulators how FICO’s AI-based AML tools work to detect money laundering.
“Sometimes we hear, ‘If I don’t work everything the old way I’m going to get a huge fine,’ ” he said. “That message hasn’t filtered down that there’s an openness there to emerging technology.”
The CFPB, the Office of the Comptroller of the Currency and the Federal Reserve all declined to talk for this article.
Regulators have taken some steps concerning AI. They issued a joint statement in December, for example, encouraging AI's use to comply with the Bank Secrecy Act and other AML rules. They specifically called out AI software as worth testing.
Some hail that as progress, but it's not clear whether that's enough. And bankers want to go beyond just using AI in AML and also use it in consumer lending, among other areas.
Merrill at ZestFinance said he has spent time with bank regulators, explaining how his company’s machine learning software works.
“We’re making a lot of progress there,” Merrill said. “There’s still a lot of work.”
Stein said he’s had several discussions with people at the CFPB about AI and the types of data the company uses in its underwriting models.
“Their reaction has always been, we’re not concerned with the technical process, we’re concerned that you’re complying with the regulations,” he said. “If someone is being declined, are you explaining to them why they’re being declined and is the model consistent in explainability?”
For instance, if a model says a public record is the reason for a decline, there had better be a negative public record in that potential borrower’s credit report.
“It’s not a black-box situation,” Stein said.
Editor at Large Penny Crosman welcomes feedback at